"How can we use technology to improve education?"
Make online learning more motivating with learning analytics
Can we use technology to better motivate students who are at risk of falling behind? Can we closely monitor learning outcomes in lessons involving large groups of students? And can we make interventions to improve learning outcomes scalable and sustainable? Finding answers to these questions was the goal of the PerActiLA project, which stands for personalised student activation through learning analytics-based insights on learning processes.
Besides lecturer, Uwe Matzat is also a researcher at Eindhoven University of Technology. His research interest is educational technology. He tries to find answers to the question: how can we use technology to improve education? In this, learning analytics obviously plays a role, he says. "Especially during covid, the need arose to know more about the learning process of students. After all, we had plenty to do with the limitations of online education and we wanted to know: which students are falling behind? And can we motivate them to spend more time on their studies?"
Predicting learning disadvantages
He therefore submitted an application to SURF for the Open and Online Education Incentive Scheme for the PerActiLA project. Matzat: "Actually, we are testing two things in this project: the contribution learning analytics can make to identifying students who are falling behind and the influence of mindset interventions on this group."
Mindset interventions consist of a combination of educational videos, reading components, personal stories from other students and writing exercises. "They are short interventions, in our case two sessions of twenty minutes, that students can do at home. That way, we don't ship students with a lot of extra work. Moreover, as TU/e, we already have quite some experience with mindset interventions. We suspected that these work well with students who are in danger of falling behind. The aim of this project is to investigate whether this is actually the case."
Although covid was the immediate reason to start working on this, the intention is of course to deploy the method in normal periods without constraints as well. "Because in large courses of undergraduate studies, it is always difficult for lecturers to find out which students are keeping up or not. After all, you have a hundred students or more in front of you; you can't supervise them all individually. We therefore also wanted to learn how to use learning analytics to quickly find out which students need some extra attention," he says.
"We wanted to learn how to quickly figure out which students need some extra attention based on learning analytics."
Complicating factors
Although the idea is simple in theory, working it out in practice is difficult, Matzat knows. And for a number of reasons. "First of all, you have to involve all stakeholders: students, lecturers, other researchers, IT people, the legal department. It takes a lot of time to get all stakeholders on board and make them understand why we are doing this."
In addition, privacy is a concern. Matzat: "Of course you have to comply with the GDPR, but that's not all. Because a data protection impact assessment (dpia) is nice. But you also - and actually mainly - have to make sure that students understand what they gain from the mindset interventions. If they see the benefit of early guidance, they will be more inclined to consent to the use of their data than if they think we are just using that data for ourselves."
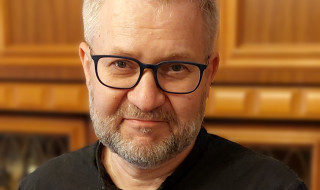
Finally, extracting insights from the "huge bucket of data", as Matzat calls the data in Canvas' Learning Management System (LMS), was not so easy. "We had so much data at our disposal. The question is how to convert that data into actionable insights. We wanted to predict which students are likely to fall behind as early as four weeks after the start of a course. The question is: which data are predictive and which are not? To find out, we tried different models and different datasets. Sometimes we found that the data needed some processing before it was usable. With other datasets, we discovered that they had no predictive power. It really is puzzling; trial and error. We found out, for instance, that the design of a section played a much bigger role in the predictive value of data than we had thought beforehand. So you then have to consult very carefully with lecturers about how their course is designed."
"This way of increasing learning outcomes is not reserved for technical subjects. You can apply it in all subjects that you teach to large groups at the same time."
Results
After four weeks, the team led by Matzat unleashed the mindset interventions on the students. All students received the same interventions, so that the team could properly analyse afterwards whether the impact of these interventions was greater among students at risk of falling behind. It turns out that it works relatively well to predict how successful a student is going to be in the subject after only four weeks. Mindset interventions have also been shown to be a good way to increase student engagement. However, it does turn out that the differences in impact between weak and strong students are less than previously thought. Matzat: "We expected weak students to benefit relatively much more from mindset interventions than strong students. We want to tinker with this further, to study precisely the effect in weak students even further. After all, the aim is to help them early on."
Besides these direct results, there are also many indirect effects, Matzat explains. "For example, cooperation with the IT department has improved. They understand better what we want to do with learning analytics, which means they can also start to inspire and advise us in the future."
In addition, there is a lot of interest from the three other technical universities in our country that TU/e collaborates with in the 4TU Federation. "They also want to work with learning analytics and they are going to use our project results to do so," Matzat says not without pride. He also already sees cautious interest from other universities. "Because this way of increasing learning outcomes is not reserved for technical subjects. You can actually apply it to all subjects that you teach to large groups at the same time."
Work together
Precisely because learning analytics and educational interventions have such broad applications, Matzat urges others working on the same topics to get in touch. "I see that currently many different places are working on applications and techniques that are in line with each other. If we act together, we won't have to reinvent the wheel in more places at the same time; instead, we can build on each other's results and thus gain more speed together."
Are you working on learning analytics and/or mindset interventions? And would you like to learn from others? Then sign up at u.matzat@tue.nl
More about the Open and Online Education Incentive Scheme
This project was created with help from the Open and Online Education Incentive Scheme. View more results from the stimulus scheme projects.